Artificial Intelligence will be an accelerant: For productivity, collaboration and efficiency. But only, when applied with focus. For companies it is time to calibrate their business models to an AI future.
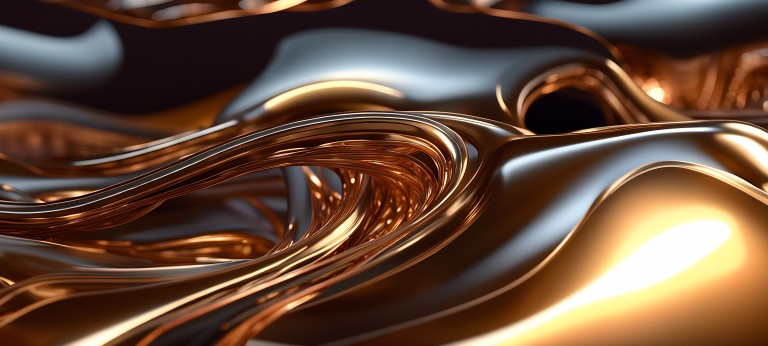
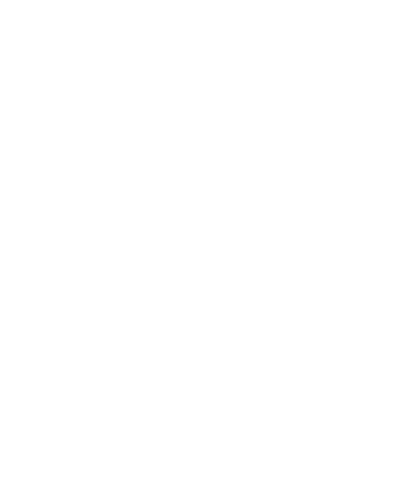
Generative AI - A way out of the productivity crisis?
By Jochen Ditsche and Fabian Neuen
How companies can harness the potential of generative AI
Hopes are high for generative AI. It is expected to do nothing less than resolve the productivity crisis in advanced economies. A new study by Roland Berger shows that these hopes are well founded. If properly applied, language models like ChatGPT can open up completely new possibilities for increasing productivity – such as by taking over routine back-office tasks or helping people with complex parts of their job as a digital co-worker.
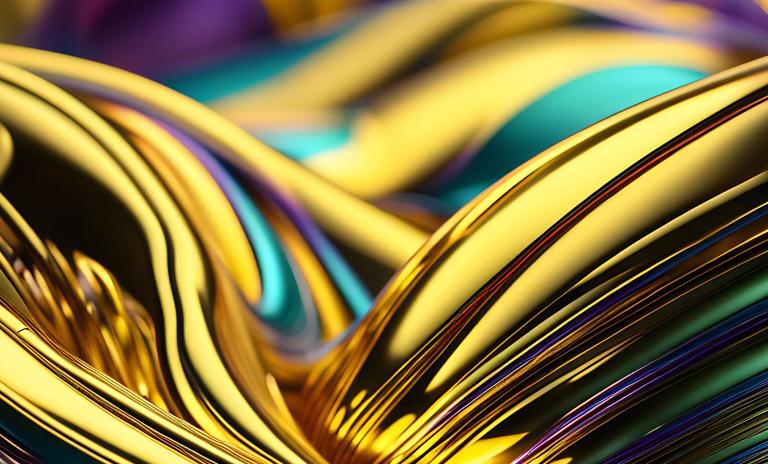
Interestingly, the use of the new AI language models will not lead to any significant reduction in companies' headcount for the time being. Only 16% of the executives surveyed in the study plan to cut jobs by 5% or more by the end of 2025. The majority expect to see no change at all. When it comes to the anticipated productivity gains, the situation is the exact opposite: The vast majority of respondents (82%) consider an efficiency gain of at least 6% from generative AI to be realistic.
The current sentiment among executives is consistent with experience from previous waves of innovation. Historically, it has been shown that major technological advances create more new activities than they destroy in the long run. This makes reskilling and upskilling all the more important going forward.
That said, there are some striking differences in the extent to which companies are making use of the new tools in practice. While some industries are forging ahead, others are still experimenting very cautiously with generative AI . The frontrunners in our rankings are wholesale and retail; information and communication; professional, scientific and technical activities; and other service activities. In all of these sectors, at least 57% of companies have already deployed generative AI tools in their organizations. In traditional manufacturing, by contrast, only 14% of companies are currently exploring the potential of generative AI.
A similar disparity can be observed across industries in the different corporate functions. While the new tools already play a role in everyday life in marketing and communications or customer service, their use has been very sporadic so far in logistics or legal departments. This is despite the fact that it would not be difficult to identify use cases within these functions as well. For example, AI language models could take over research into legal precedents, write first drafts of contracts, or perform repetitive due diligence checks.
Something that should not be ignored is the effect of generative AI on job quality. Generative AI has the potential to increase how well employees identify with their job, and thus enhance people's job satisfaction. This will be the case if the tools leave more time in the day for meaningful "human" activities. Such activities could, for example, involve deepening customer relationships or structuring strategic issues of fundamental importance.
Despite the current investment boom, a whole series of technical and practical hurdles still stand in the way of the widespread application of generative AI. These include privacy issues, such as the protection of trade secrets and personal data, or the fact that the current generation of language models freely invents answers on occasion (referred to as "hallucination"). The same goes for successfully embedding generative AI applications into organizational workflows, which many companies find difficult to achieve in practice. Only once these hurdles have been overcome will companies be able to exploit the full potential of the new technology.
Three steps to successfully implementing generative AI
Analyze the impact of AI
To develop a solid AI strategy, companies first need to analyze their entire value chain to determine what efficiency gains can be realized through generative AI at which stage. We recommend using the "Roland Berger AI Readiness Radar" to do this. The findings should then be benchmarked against competitors' AI adoption and general industry trends, flanked by an analysis of the company's current IT infrastructure.
Define your AI strategy
Once this inventory exercise is complete, we advise clients to define overarching goals and productivity benchmarks. The next step should then be to plan the implementation of initial use cases. Improving the management of all unstructured data sets is of critical importance here. All process data must be prepared in such a way that established models can be fine-tuned to the given business purpose. Alternatively, it must be possible to develop special-purpose models from them for very specific tasks.
Establish proof of concept
Once all the relevant technical details have been determined – such as selection of the AI model, the vendor, and the integration plan – the implementation of the first use case can begin. This will be followed by an evaluation, the iteration of any improvements and, lastly, a company-wide rollout plan. The overarching goal for any company should always be to make the whole of its organizational knowledge available via its own generative AI model or even a modified off-the-shelf model.
Register now to download the complete study with all the insights on how companies can harness the full potential of generative AI. Additionally you get regular insights and news directly into your inbox.