" Nature isn't classical and if you want to make a simulation of nature, you'd better make it quantum mechanical. "
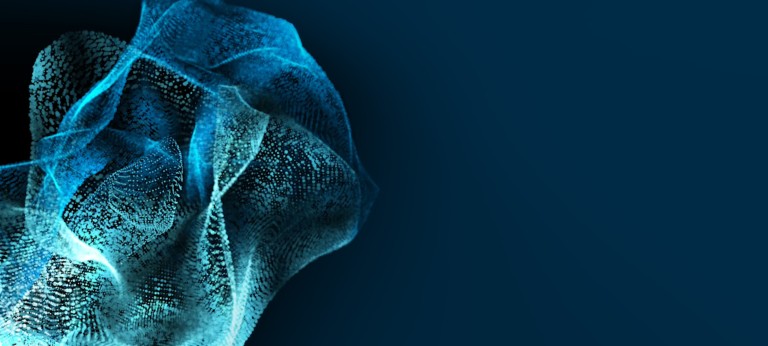
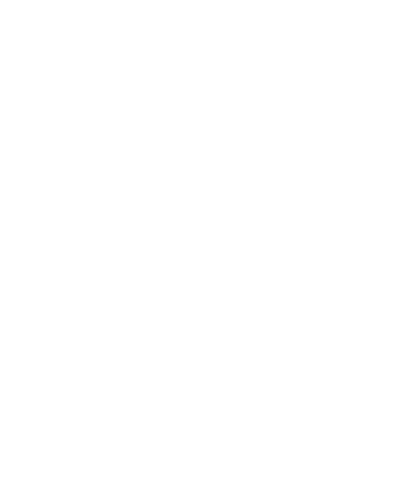
The next big thing: Quantum machine learning
It's been a quantum leap into 2018. Intel and IBM both revealed their new generation of quantum computers at the CES conference in Las Vegas. A team of physicists from University College London published a breakthrough study on creating a hack-proof quantum internet, showing that completely secure communications are possible. And encrypted images have been transmitted long distance – from Beijing to Vienna – for the first time ever via a quantum network. All in the first month of 2018!
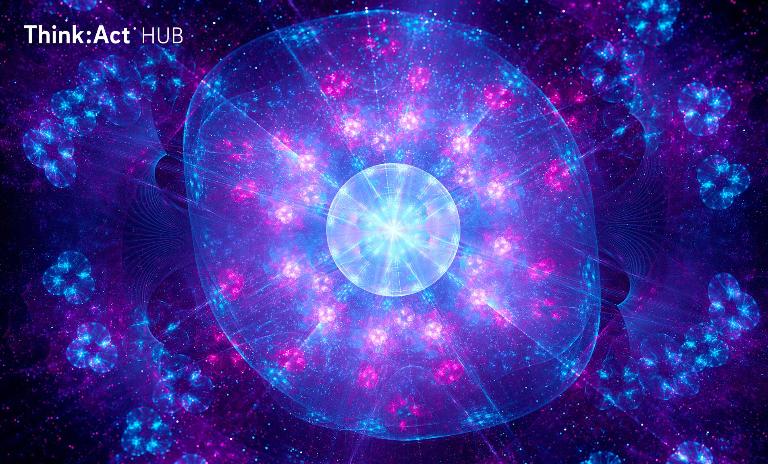
Challenges in machine learning lend themselves particularly to quantum computing: "QC will play a critical role in the creation of artificial intelligence," says Geordie Rose, Founder of D-Wave, one of the first companies to build quantum computers. The MIT tech review agrees: "Quantum computers will be particularly suited to factoring large numbers […], solving complex optimization problems, and executing machine-learning algorithms. And there will be applications nobody has yet envisioned." Clearly, quantum machine learning (QML) is going to be the next big thing, disrupting the already mind-boggling field of artificial intelligence.
As a theoretical physicist, I am thrilled by this development. I vividly recall my doctoral exam about twenty years ago, when I was asked about the potential impact of quantum computing and whether this theoretical idea would ever make it into the real world. At the time it was impossible to say. Ever since, I have been following developments in this exciting field and I now feel privileged to witness quantum computing approaching the mainstream.
At the same time, as a business leader and consultant, I have been asking myself to what extent businesses need to be worrying about this topic now. Is it just another technological step on the path to the commoditization of practically unlimited computing power? After all, we seem to get on perfectly well without knowing all the ins and outs of how the silicon chips work in our computers today. Yet quantum computing appears too fundamental to ignore. It makes sense to at least embrace this field to the extent that you understand the new developments and can assess their potential impact on business somewhere down the road.
On the Gartner Hype Cycle for Emerging Technologies, quantum computing is currently considered an "innovation trigger". It will take more than ten years until its mainstream adoption. However, I believe that that timeframe is potentially misleading. A more nuanced assessment is required.
While general-purpose quantum computing is indeed broadly expected to be a decade or more away, special applications may be just a few years off. Machine learning happens to be one such application, advancing the already hot field of artificial intelligence.
In any case, getting our head around what it's all about seems to be a good place to start.
Quantum computing enables exponential increases in speed by harnessing the weirdness of quantum mechanics. The key challenge is to build robust systems at scale.
It was back in 1982 that Richard P. Feynman first suggested the idea of quantum computing. "Nature isn't classical and if you want to make a simulation of nature, you'd better make it quantum mechanical," he wrote. Feynman claimed that classical computing is not suitable for modeling complex molecules. This still holds true today. For example, pharmaceuticals can only be tested by means of risky, real-life testing, not by simulations.
"Quantum computers will play a critical role in the creation of artificial intelligence."
Feynman went on to say that quantum computing has the potential to broadly outperform classical computing. Why? Because in quantum mechanics everything is possible at the same time, while in the classical computing world everything is binary.
The key unit of quantum information is the qubit ("quantum binary digit"). A classical bit can be in one state, either 1 or 0, whereas a qubit can be in two states simultaneously – a concept known as "superposition" in quantum mechanics. Thus, a string of two qubits can be in up to four states simultaneously (<00>, <01>, <10>, and <11>), each with a certain probability. Two classical bits can only represent one of those four states at any given moment. In general, n qubits can be in two to the power of n states, as opposed to just one state for a string of n bits. This is what makes quantum computing faster than classical computing. It is expected that n in the magnitude of around 50 will be needed to outperform classical computers for general purposes.
However, that is only if the qubits keep their quantum properties perfectly. Clearly, quantum mechanics goes beyond anything we can imagine. Quantum effects are averaged out by thermal fluctuations, radiation and the sheer quantity of particles making up everyday objects. Preserving quantum behavior at scale is a massive undertaking. In 2012 Serge Haroche and David Wineland won the Nobel Prize for Physics for inventing ways to trap and manipulate particles while maintaining their quantum properties. This basically requires keeping particles away from heat and radiation, which is why such heavy machinery needs to be built around chips today. Particles need to be kept in a vacuum at temperatures far below -200 °C (-420 °F).
Many challenges must still be overcome before quantum computing becomes mainstream and delivers on its full potential. Research is ongoing in two areas. First, dealing with probabilistic states requires new ways of coding and new algorithms for processing information. Second, fundamental progress is needed on building robust quantum systems at scale – systems that can hold sufficient amounts of qubits under suitable conditions, such as on a silicon chip at room temperature.
Many players are involved in different areas of development. Initial tests on hybrid models are already up and running, using QML to enhance classical computing.
Achieving quantum supremacy still requires significant progress in a number of areas. Many different players are involved, most of them specializing in specific pieces of the overall puzzle.
As far as the number of qubits goes, fully functioning quantum computers have been built with four to five qubits, while fragile test systems reach ten to twenty qubits. The prototype presented at CES by IBM this month has 50 qubits, while that of Intel has 49 qubits.
These achievements have drawn much attention, as around 50 qubits is the theoretical threshold for quantum computers to outperform classical computers for general purposes. However, this is somewhat misleading, as the theoretical threshold assumes perfectly robust qubits. Taking into account error rates and the difficulty of maintaining robust quantum properties, the required number of qubits under real-life conditions could be a few hundred or even a few thousand. In many ways the race for qubits is reminiscent of the race for transistors half a century ago.
Microsoft and Google have set up general-purpose quantum computing R&D programs but not yet publicly demonstrated their hardware. Google has been running its Quantum AI Lab together with NASA and the Universities Space Research Association since 2013. The company says that it is "particularly interested in applying quantum computing to artificial intelligence and machine learning. This is because many tasks in these areas rely on solving hard optimization problems or performing efficient sampling." Microsoft has been especially active in developing a programming language for quantum computing based on C#, as well as enabling easy access through Azure. The company's focus seems to be on making quantum computing accessible to developer communities.
Alongside these tech giants, Berkeley-based startup Rigetti Computing is driving the commercialization of QML. The company emerged from Y Combinator as "space shot" and is backed by big names in the tech space, such as Andreessen Horowitz and Vy Capital. The firm is already running unsupervised machine learning on its quantum computer system based on clustering algorithms.
IBM and Rigetti have also both introduced capable general-purpose cloud-based quantum computers for public and limited-access use. IBM's is a 20-qubit system and Rigetti's a 19-qubit system. Each system comes with a full-stack software development toolkit. IBM's Q Network aims to explore potential practical quantum applications based on its current 20-qubit system. JP Morgan, Daimler, Honda, Samsung and Volkswagen are reported to be among the first clients.
Ironically, while the general perception is that quantum computers make the world an unsafe, hackable place, the promise of excellent safety for sensitive information is also the very thing that drives research. The aim is to enable hack-proof communications via the so-called "quantum internet". The United Kingdom and European Union have recently launched joint research projects to establish the hack-proof transfer of information between major European cities based on quantum networks.
The key promise for business is that quantum computing will be able to extract the maximum meaning from Big Data. Generally, players are keeping relatively quiet about their achievements here. Tech giants such as Alibaba and Tencent are among those generating least noise. Startups such as IonQ, Quantum Circuits and RIKEN are also increasingly investing in the development of hardware. However, none of these players has shown their work publically yet.
QML will advance artificial intelligence to an unimaginable extent. Businesses should be aware that all data-related problems will be solvable very soon.
For the time being it is still difficult to predict exactly when specific applications of machine quantum learning will become relevant for mainstream business. However, there are signs that we are looking at a few years rather than a decade or more. That means that disruptive progress in extracting meaning from data will happen within what many businesses consider their strategic time horizon.
Businesses should not wait for QML to hit the mainstream. As with all exponential developments, the tipping point will come suddenly after periods of slow progress. Companies need to embrace now the vision that all data-related challenges will be able to be resolved easily at some point in the next few years, and rework their value propositions and operating models accordingly.
It will be crucial to follow closely developments at the companies involved. The impact of quantum computing will be so great that whoever gets their hands on new solutions first will vastly outperform their competitors. This is also why companies are communicating about their progress in this field much more cautiously than about other emerging technology.
Given the current momentum on this topic, I expect that we will find out more about what to expect – by when and from whom – in the next few months. Stay tuned!