Our consulting services offer you a range of future-oriented solutions that support your company to transform your manufacturing approaches towards Next Generation Manufacturing.
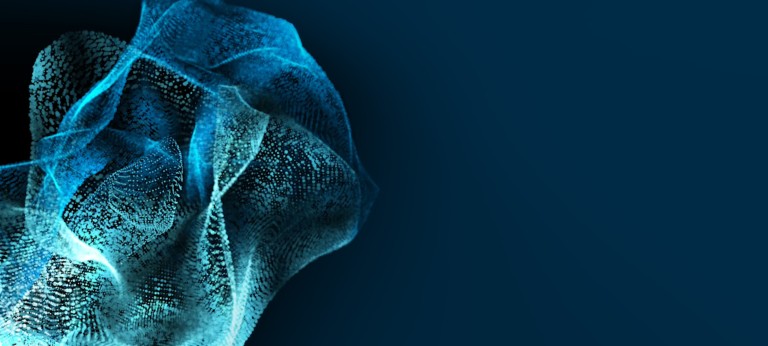
_download_preview.jpg)
Whatever happened to "Industry 4.0"?
How automotive players are leading the way on digital manufacturing – and what other industries can learn from them
Since its inception over a decade ago, the German concept of "Industrie 4.0" – referencing the tremendous advances promised by digitalized manufacturing operations – has come a long way. In many areas, the automotive industry has taken the lead. So, to separate hype from reality, Roland Berger recently conducted in-depth interviews with top global automotive OEMs, Tier-1 suppliers and other industry experts. Summarizing an exhaustive Roland Berger report, this article ultimately distills valuable lessons that other industries can learn from automotive’s "Industry 4.0" journey to date.
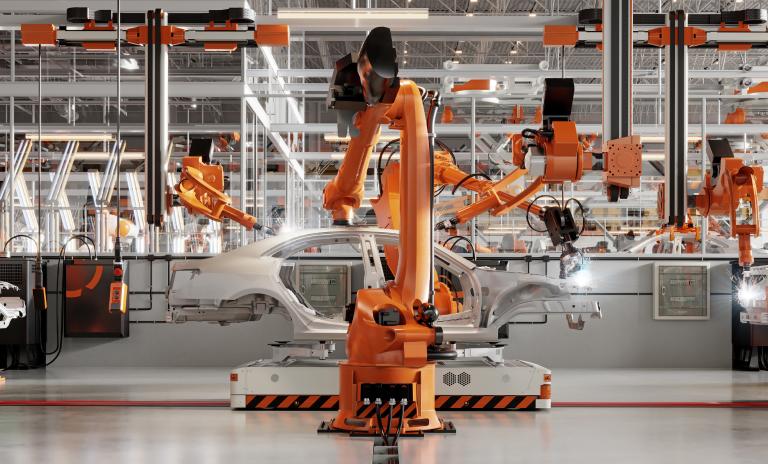
"As different processes and/or technologies in automotive production are more or less suitable for digitalization, weighing up how much added value can actually be created is a very important factor for each individual use case."
A decade of digitalized manufacturing
A good ten years ago, the (German) automotive industry in particular began investing heavily in identifying, developing and rolling out use cases for digital manufacturing. When the initial euphoria faded, it encountered numerous technological and organizational challenges, including heterogeneous and legacy infrastructures, problems quantifying the benefits of digital use cases (and hence onboarding investors) and the need to retrain and or recruit suitably qualified staff. Also, intracompany silo mentalities often stood in the way of the cross-functional cooperation that was now needed.
More than a decade later, the original vision of autonomous, highly flexible and self-organizing factories is still way off in the distance. In order to identify where "Industry 4.0" is really at today, Roland Berger therefore dug deeper, tracing what has (and has not) happened, examining those use cases on which the automotive industry has focused, how far they have progressed, where differences exist between OEMs, OESs and varying regions, and exploring how these use cases are being developed and implemented – along with necessary organizational changes – in practice.
What does the car industry use "Industry 4.0" for today?
Identifying seven key use case “archetypes” in the automotive industry, Roland Berger found that five specific use cases – subsets of these archetypes – have so far been prioritized. These are: real-time machine parameter optimization; condition monitoring and predictive maintenance; automated vision-based/process data-based inspection; autonomous material handling in intralogistics; and the tracking of transports along the supply chain.
"The number of use cases for cobots with a positive business scenario is limited. Cobots are mostly only suitable for post-automation of workstations that were originally designed for manual work."
The fact that cobots do not appear on this list is perhaps equally revealing. After the hype has passed, the number of use cases for cobots with a positive business case have turned out to be limited. Nowaday, cobots are mostly used for post-automation of workstations that were originally designed for manual work.
Since different processes and/or technologies in automotive production are more or less amenable to digitalization – which will doubtless also be the case in other industries, too – weighing up how much value can genuinely be added is a very important factor for each individual use case. Roland Berger therefore took a deep dive into the manufacturing processes used by OEMs and OESs, as well as examining how differences between low-cost and high-cost countries, for example, can affect companies’ digital priorities. Five high-priority use cases for automotive players highlight the benefits and the challenges involved. The brief summary provided below is explained in considerable depth in the full report:
- Condition monitoring and predictive maintenance
An obvious choice given its potential to ensure higher availability and deliver better overall equipment effectiveness (OEE), predictive maintenance has in practice encountered several implementation challenges such as expensive retrofitting or fixed maintenance schedules anchored in automotive companies. As a result, only fairly simple predictive maintenance applications (e.g. motor current analysis) are actually being rolled out at the present time. More complex use cases that cover complete systems (e.g. industrial robots) are still under development. Despite these challenges, the substantial value of predictive maintenance, especially when implemented in large-scale systems such as CNC machining centers in component manufacturing or industrial robots in body shops, and the amount of resources invested indicate that the number of predictive maintenance use cases is set to increase sharply in the years ahead. - Automated quality inspection
Process and sensor data can be used to detect or predict potential quality issues by identifying anomalies in the data. It can also be deployed to replace spot checks with data-based inspections and even add more, earlier and more accurate quality checks. A distinction is drawn between vision-based and process data-based inspection:
Vision-based inspection of single parts, inline checking and early checking are the main areas of focus here. Deep machine learning has brought major improvements, allowing vision-based quality inspection to add considerable value. Broadly speaking, the technology is mature enough to be implemented quickly. However, most use cases are still in early stage/pilot implementation or even under development. In the years to come, the ability to use cheap cameras with complex machine learning models will fuel wider rollouts.
Process data-based quality inspection: Again, simple applications (verifying the quality of screwed parts, for example) are the easiest to implement thanks to the many models and process controls that are already in service. Where well-understood processes and parameters are used in conjunction with simple statistical models, deployment certainly yields tangible benefits. Overall, however, this approach is still less mature than vision-based alternatives. More complex variants are still being researched. - Real-time machine parameter optimization Attempts to replace the rare and expensive process experts who normally set machine parameters are obviously appealing, so automated optimization is already used to adjust process parameters in clean room management, for instance. Accordingly, very rudimentary uses cases, again based on selected parameters for fully mastered processes, are already being rolled out. Here too, however, a raft of challenges mean that more complex use cases are unlikely to be implemented in the next decade. For the time being, machine parameter optimization in the automotive industry will thus remain restricted to information input as decision support for human process experts.
- Autonomous material handling in intralogistics
Substantial cost-cutting potential puts this kind of application high on automotive companies’ list of priorities. Automating indirect material handling (to complement direct transportation tasks that are already largely automated) could indeed yield considerable savings on labor costs and errors/scrap in production environments. The problems of varying object shapes and working spaces shared with people have not yet been thoroughly resolved, however, so autonomous transportation of large and heavy objects is not yet fully viable. On the upside, the transportation of small, lightweight and less critical components by automated guided vehicles is already working well, both between warehouses and assembly lines and in outbound logistics. - Tracking of transports along the supply chain
After initially seeking to surmount early challenges by focusing on inbound logistics for critical components, some OEMs are now moving onto tracking outbound deliveries of finished vehicles. Automated real-time tracking can offer significant rewards in a context where production outages are hugely expensive, so this use case is understandably coveted by many automotive players.
How is the automotive industry driving development and implementation?
Roland Berger talked to automotive industry leaders and experts about ways to deal with the many challenges and hurdles touched on above. The resulting report identifies similar patterns and blueprints in two key areas: in organizational setups and operating models on the one hand, and in IT/OT architectures on the other.
- Organizational setups and operating models
To overcome the redundancies and discrepancies born of traditionally independent manufacturing plants, larger digitally minded OEMs in particular tend to favor hub-and-spoke organizations: Adopting a largely use case-driven approach, central units pool the required digital and production skills and liaise closely with (decentral) production plants. Most organizations operate a five-step model to identify and prioritize attractive use cases, develop and pilot prototypes and then roll these out across the production network. The full Roland Berger report goes into more detail on the kind of communication and cooperation that is needed between the "hub" and the "spokes" to make this model work efficiently.
- IT/OT architectures
Putting an overarching IT/OT architecture in place helps automotive players avoid the redundancies and mismatches that can easily occur when use cases are rolled out one at a time. Standardization and interoperability are built into these architectures from the outset, allowing new use cases to be rolled out swiftly, efficiently and coherently. Leading German OEMs are already seeking to integrate Tier-1 suppliers in these architectures, whereas OESs are currently focusing more on internal harmonization.
What can other industries learn from automotive’s approach?
A wide range of industries can learn valuable lessons from the pioneering work automotive players have done and continue to do in the arena of digital manufacturing. Roland Berger’s in-depth report condenses its findings into four practical recommendations for companies who want to carry "Industry 4.0" over into other sectors:
- Prioritize use cases and resources
Quantifying the value that can be added in terms of cost savings, quality gains, energy savings or whatever enables companies to identify and focus on those use cases that will deliver the greatest returns. This in turn creates a convincing business case that will obviously vary depending on the economic environment and regional factors. - Set up a hub-and-spoke organization
A central unit (the "hub") must combine data analytics and other production and IT-related capabilities with the authority to initiate change in decentralized production plants (the "spokes"). Conversely, the plants must buy into what the center is doing, so transparency and compelling, two-way communication are essential. The value added by the digital manufacturing unit must be clear for all to see. - Redesign training and talent recruiting
Existing approaches to both training and recruiting must be aligned with the emerging need for digital and data analytics skills, the requirement for operators to work alongside automated equipment – and the importance of getting regional leaders and plants to buy into digital manufacturing use cases. - Formulate a target IT/OT landscape and roadmap
Once initial use cases are delivering results, an IT/OT target landscape – and a roadmap showing how to get there – is vital to exploit the full potential of digital manufacturing without wasteful overlaps and redundancies in the long term.
For a much more in-depth discussion, complete with a wealth of practical guidance and examples, you can read the unabridged Roland Berger report. Alternatively, mail us if you have any questions, comments or suggestions. We value your input and look forward to hearing from you.
Register now to download the full study on “Whatever happened to Industry 4.0” including key insights, an outlook on the developments of the automotive industry as well as use cases for respective players within the industry. Additionally, you'll get regular insights into automotive topics.